What is the difference between bias and variance in the context of model evaluation?
In the world of machine learning, attaining the best performance of a model requires a delicate balance of two fundamental concepts such as bias as well as variance. The concepts of bias and variance are crucial aspects of evaluating models that affect the generalization and accuracy capacity of a machine-learning model. In this post we will explore the subtleties of variance and bias by exploring their definitions, impacts on model performance, as well as methods to find the perfect balance. https://www.sevenmentor.com/data-science-course-in-pune.php Definition of Bias and Variance: Bias: Bias refers to the error that is introduced by attempting to approximate an actual-world issue using an abstract model. Algorithms tend to always diverge from actual values. A high bias suggests the algorithm is simplistic and could miss out on intricate patterns in the data, which can lead to overfitting. Variance: Variance, on the other hand, measures the model's sensitivity changes in the data used to train. It is a measure of how the model's predictions could differ if it was trained on a different set of data. A high variance means it is excessively complicated and can detect errors in the training data which results in poor generalization to data that is new and untested which is referred to as overfitting. Impact on Model Performance: Bias: High-bias models typically produce mistakes that are systematic and often misrepresent the fundamental patterns of the data. The models that aren't well-fit fail to grasp the complexity of the data and result in low performance both on the testing and training sets. Variance: High-variance models are likely to become too flexible and can capture errors in the training data but fail to adapt well to new data. Overfit models are successful in the training set but are unable to perform well in the test set because they are unable to expand beyond specific examples found in their training datasets. The Bias-Variance Tradeoff: The best model is finding the perfect equilibrium between variance and bias because these two variables tend to conflict with one another. This is essential when creating models that can be generalized to data that is not seen. Finding the right balance, it requires changing the level of complexity of the model and improving the parameters of hyperparameters. Strategies for Managing Bias and Variance: Model Complexity: A higher degree of complexity in the model can aid in reducing bias, and allow the model to recognize more intricate patterns within the data. It is crucial to keep track of and manage the complexity of the system to prevent overfitting which can cause more variance. Data Science Classes in Pune Regularization: Regularization techniques, like L1 and L2 regularization penalize models that are too complex aiding in limiting variation and preventing overfitting. Cross-Validation: Cross-validation is a reliable method for evaluating model performance. It works by breaking the data down into validation and training sets several times. This can help to identify and address issues related to variance and bias. Ensemble Methods: Ensemble techniques, such as bagging and boosting, blend the results of several models to enhance overall performance. These techniques help to strike an equilibrium between variance and bias. Data Science Training in Pune Conclusion: In the tangled world of machine learning understanding and managing the effects of variance and bias are crucial in developing models that adapt effectively to new information. The tradeoff between bias and variation is the basis for guiding principles, stressing the importance of an approach that is balanced to model the complexity. By carefully altering the parameters of the model by incorporating regularization techniques and making use of ensemble methods to navigate the complicated dance between variance and bias, eventually make models that are precise and accurate predictions.
Read more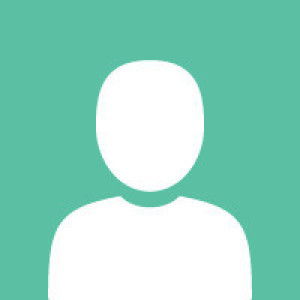
How could Salesforce be utilized to further develop client assistance and backing?
Salesforce is a cloud-based client relationship the executives (CRM) programming that helps organizations oversee and smooth out their business, showcase, and client care tasks. Salesforce can be an amazing asset for further developing client care and backing, as it gives a concentrated stage to overseeing client communications, following client issues, and settling client issues. In this article, we will investigate how Salesforce can be used to further develop client assistance and backing. Unified Client Information One of the greatest advantages of Salesforce is its capacity to unify client information. Salesforce stores all client data, including contact data, buy history, and backing connections, in a solitary data set. This makes it simple for client assistance specialists to get to the data they need to give viable client service. With Salesforce, specialists can quickly see a client\’s past Cooperation and buy history, empowering them to give more customized and compelling help. Astute Steering Salesforce\’s keen steering capacities empower organizations to course client requests to the right specialist, in light of variables, for example, the client\’s area, issue type, and language inclination. This guarantees that client requests are taken care of by the top specialist, prompting quicker goal times and higher consumer loyalty rates. Mechanized Help Salesforce\’s robotization abilities can be utilized to computerize dull help undertakings, for example, responding to habitually got clarification on pressing issues, sending request affirmation messages, and refreshing client records. Via computerizing these undertakings, organizations can let loose their care staff to zero in on additional perplexing issues and offer more customized help to clients. Self-Administration Choices Salesforce\’s self-administration choices permit clients to track down replies to their inquiries without reaching client service. Organizations can make an information base of articles and instructional exercises, which clients can access through a self-administration entryway. By giving clients self-administration choices, organizations can diminish the quantity of help requests they get, prompting more limited stand by times and quicker goal times for clients who truly do have to contact support. Omnichannel Backing Salesforce empowers organizations to offer help across various channels, including email, telephone, visit, online entertainment, and SMS. This permits organizations to meet clients where they are and offer help through the channel that the client likes. By giving omnichannel support, organizations can further develop consumer loyalty rates and diminish client beat. Client Criticism Salesforce\’s client criticism abilities empower organizations to accumulate input from clients and utilize that input to further develop their help tasks. By gathering criticism after each help cooperation, organizations can distinguish regions for development and do whatever it may take to address client concerns. This can assist organizations with further developing their help tasks and increment consumer loyalty rates after some time. Investigation and Revealing Salesforce\’s examination and announcing capacities empower organizations to follow their help execution and distinguish patterns and examples in client conduct. Organizations can utilize this information to settle on informed conclusions about how to further develop their help activities, for example, recognizing regions where they need to further develop specialist preparing or executing new self-administration choices to lessen support requests. All in all, Salesforce can be an integral asset for further developing client care and backing. By incorporating client information, giving keen steering, robotizing support errands, offering self-administration choices, giving omnichannel support, gathering client input, and giving examination and detailing capacities, organizations can give quicker, more customized help to their clients, prompting higher consumer loyalty rates and lower client beat. Also Read- https://www.sevenmentor.com/salesforce-training-in-pune.php
Read more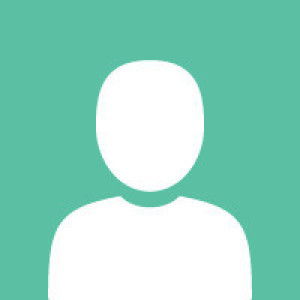
What are some common German expressions and phrase
Here are some common German expressions and phrases that you should know: 1. Guten Morgen / Tag / Abend - Good morning/day / evening 2. Wie geht es dir? - How are you? 3. Mir geht es gut / schlecht - I'm doing well / poorly 4. Danke - Thank you 5. Bitte - Please / You're welcome 6. Ja / Nein - Yes / No 7. Entschuldigung - Excuse me / I'm sorry 8. Ich verstehe nicht - I don't understand 9. Ich spreche kein Deutsch - I don't speak German 10. Wie spät ist es? - What time is it? 11. Auf Wiedersehen - Goodbye 12. Bis später - See you later 13. Prost! - Cheers! 14. Alles Gute! - All the best! 15. Vielen Dank - Thank you very much https://www.sevenmentor.com/german-language-classes-in-pune.php
Read more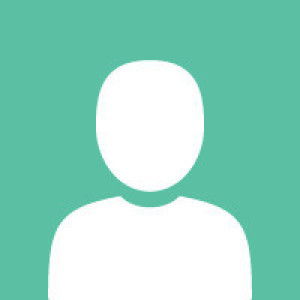